Student Colloquium: Application of Graph Neural Network in Material Science, v/ Truong Phong Nguyen
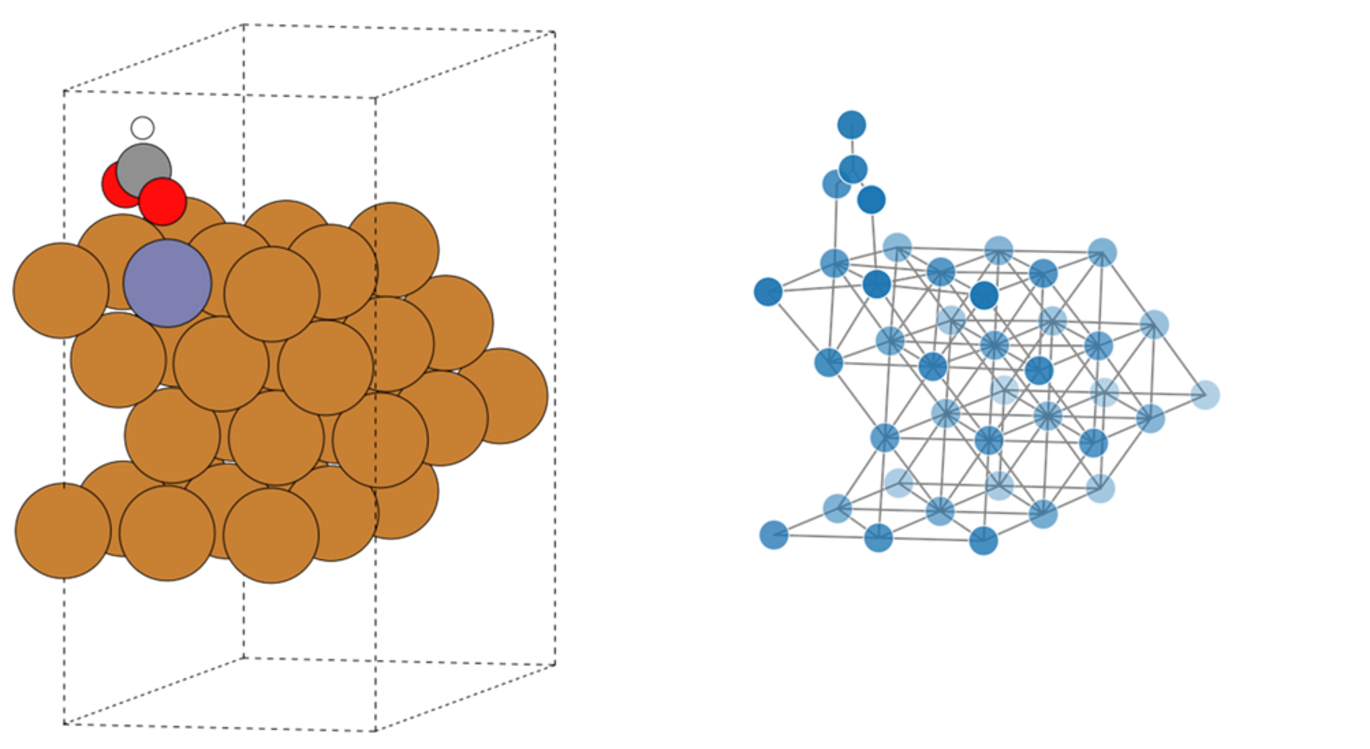
Supervisor: Raffaele Cheula
Graph theory has demonstrated its applications in a wide range of problems, including traffic optimization, social networks, and recommendation systems. Graph Neural Networks (GNNs), which are capable of predicting graph data, have revolutionized the use of graph theory in material science, providing powerful tools to model and predict complex relationships in material systems. In this presentation, we will discuss graph theory, covering foundational concepts and terminology essential for understanding graph-based representations of materials. Then, we will explore the inner workings of GNNs, which enable efficient learning and prediction for graph-structured data. Also, the catalytic activity project with zirconium dioxide (ZrO₂) dataset will be mentioned as an application. We tried to apply GNN-based model to the dataset and achieved high accuracy energy prediction with significant advantage of speed compared to traditional DFT.